-Areas where AI is more important to banks: Risk Management, Fraud and Cybersecurity, Compliance, Lending
-Fraud and Cybersecurity: AI makes fraud detection faster and more cost-effective for banks.
-AI software can get a more accurate and faster credit rating for each customer.
Artificial Intelligence For Risk Monitoring in Banking
Investment in AI by banks and financial institutions for risk-related functions such as fraud and cybersecurity, compliance, and financing and loans has grown dramatically in the last half-decade compared to customer-facing functions.
We recently launched our AI in Banking Vendor Scorecard and Capability Map report, our largest report on the state of artificial intelligence in banking. During the course of our research, we worked with experts including PhDs in AI and corporate heads of AI at top US banks to categorize AI vendor products based on the banking functions into which they are applied. We found that many of the AI products we categorized could be applied to more than one business function.
In addition, we explored the AI capabilities that make AI use-cases in various banking functions possible. In this article, we discuss the risk monitoring capabilities of AI in banking.
In the figure below, we show the number and percent of AI-based risk monitoring applications across AI vendor product offerings in banking. This is a slice of the full AI capability map:
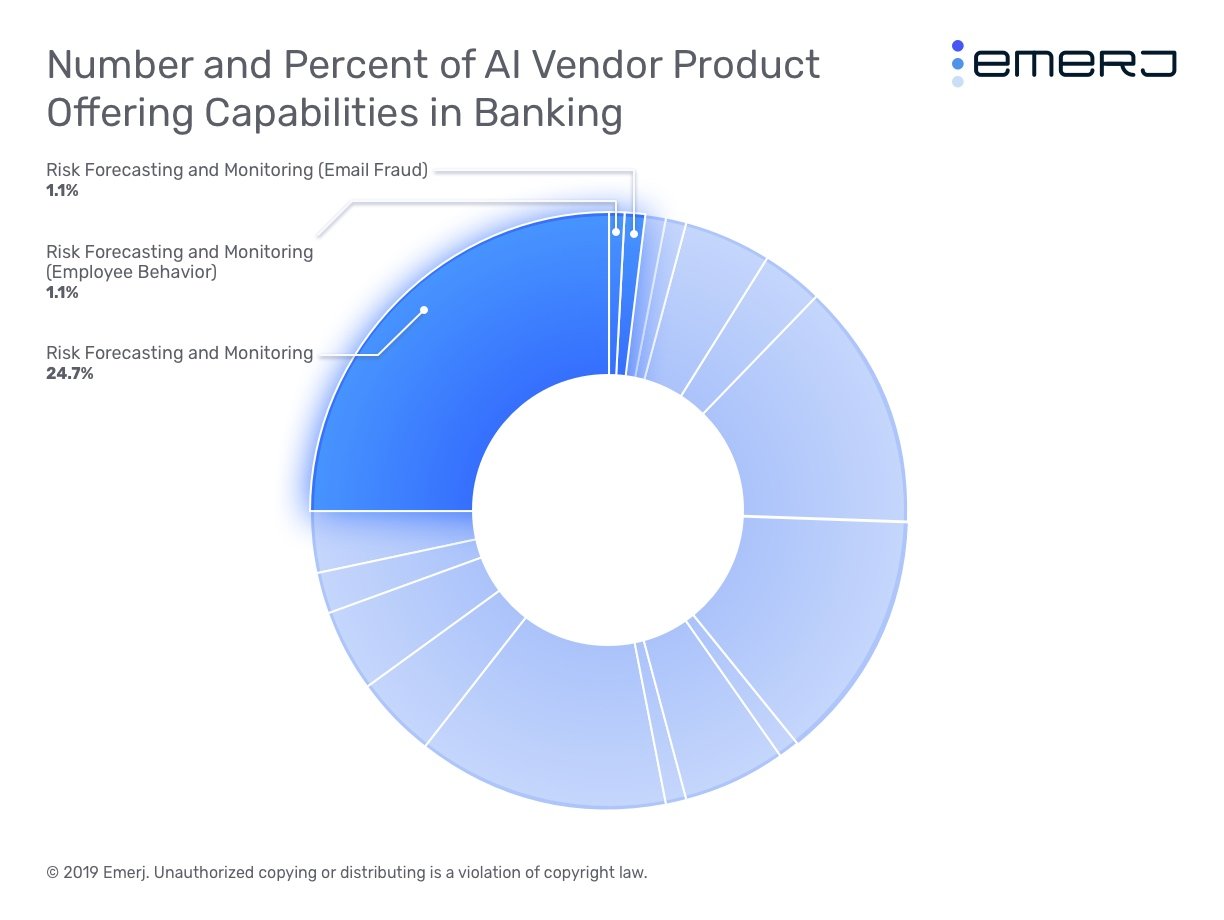
Risk Forecasting and Monitoring and related capabilities make up 26.9% of vendor product offering capabilities. The largest slice of the AI capabilities in banking is broad Risk Forecasting and Monitoring.
This data is in accordance with our interviews with banking experts who told us that bankers are focusing their automation efforts primarily on risk-related functions, in particular:
- Risk Management (trading and investment decisions)
- Fraud and Cybersecurity
- Compliance
- Lending
In this article, we explore all three of these banking functions and discuss how AI is making an impact within them and the broader risk monitoring processes at banks. We further delve into AI vendor companies serving each of these banking functions.
Our analysis begins with AI applications for risk management in banking:
Risk Management
For the purposes of our report, we largely considered AI applications for trading and investment decision-making when exploring the risk management function in banking. These applications in particular deal with mining public data for insights on what to buy and sell. Using AI software, traders, investors, and financial advisors could automatically manage trading risk by studying historical trading data.
Companies like Ayasdi and Kensho provide AI software to banks and financial institutions for risk management. For instance, Kensho, an AI Vendor that was acquired by S7P global, offers financial risk monitoring and management AI software. Kensho claims their software uses natural language processing to find relationships between financial trading data, such as stock prices, portfolio performance, company financials, news, social media, and press releases.
The software then prompt traders and financial advisors with insights that help them correlate historical events to those in the present and help them predict financial markets.
FlexTrade purportedly integrated Kensho’s analytics software into its platform. As a result, Flextrade was able to generate insights for customers based on market data such as announcements about new product launches, mergers, and acquisitions.
Fraud and Cybersecurity
Banks’ AI interest is largely focused on fraud and cybersecurity because these functions usually already have existing IT teams, infrastructure, and budgets. In many cases, automation efforts are also directed into these functions.
One of the experts from the risk management space that we spoke to during our research agreed that process automation in fraud and cybersecurity applications is where a lot of AI focus is currently in the top 100 banks.
From supply chain to the internal guts of the bank—AI can theoretically be applied to clean these processes to be more seamless and optimize those processes in real time, driving down cost and improving output…which means customers get served faster.”
Although AI-use in banking is still nascent on the whole, financial crimes and AML are where some banks are currently deploying AI solutions. This is because trying to assess customer behavior based on transaction histories and other customer specific data is hard. In many cases, this data is very complex and there are lots of fraud investigators at banks working on this, making it a highly labor intensive task.
Banks seem to be investing in upgrading and overhauling traditional fraud and cybersecurity threat detection systems with AI-based ones. One reason for this massive interest in AI in this space is because anomaly detection, an AI approach used to identify outliers in a dataset, makes fraud detection faster and more cost-effective for banks.
AI systems could help automate certain processes and augment the capabilities of human fraud or security officers to screen far more threats than just human teams alone. Additionally, there is some evidence for AI systems performing more accurately than humans alone by reducing false positives.
Banks are obligated by supervisory bodies to report any instances of fraud or money laundering. Many of these cases usually involve fraudulent bank accounts. Identifying and detecting cases of fraudulent accounts and transactions for a retail bank is slow and difficult using rules-based systems because they can generate several false positives.
Additionally, for a retail bank trying to acquire new customers, managing fraud risk while simultaneously keeping customer experiences hassle-free is critical. AI software could help banks identify and detect fraud cases with higher accuracy and at larger scales.
Companies such as Darktrace and Feedzai claim to have worked with banks and financial institutions in AI projects to improve the efficiency of their processes. For instance, Feedzai claims to have worked with a top 10 retail bank to improve the bank’s customer account opening process.
According to Feedzai’s case study, the bank needed to sift through new account applications and approve new customers while simultaneously assessing risk efficiently. The company claims the bank deployed Feedzai’s AI software and successfully increased new application approvals and reduced false positives. The company also claims there were zero increases in fraud losses from any of the new customers.
Compliance
Compliance is a “dark horse” application for AI in banking. After fraud and cybersecurity, AI vendors offering products to banks and financial institutions for compliance-related use-cases received the second most overall funding. At the same time, when we looked at the AI use-cases that the top 100 banks seemed to be marketing, only 5% of all AI use-cases considered were compliance-related.
This is a phenomenon that we call the “lens of incentives.” Banks seem to be the most interested in talking about their customer-facing AI applications in press releases and media. In reality, a majority of their actual money is going into risk-related banking AI applications such as those in compliance.
As with fraud and cybersecurity, compliance in banking is a space that already has existing automation efforts in many of the top 100 banks. This is largely due to the fact that banks see compliance as a burden that doesn’t do much to help improve their bottom-lines. Given this, banks have invested in automating the business processes in this function in order to ensure regulatory compliance at a minimized cost and time.
In addition, banks are being forced to adjust to new regulatory changes such as the GDPR and the BASEL IV mandates. The evolving compliance scene means compliance officers in banks need to scour through thousands of websites and regulatory documents to ensure that the bank is meeting all the required regulations. Failure to do so could lead to fines or even losses of banking licenses.
NLP-based information retrieval software is now being deployed to assess and monitor compliance risk at banks. These types of software can help compliance officers at banks scour through vastly more volumes of regulatory data than they might have been able to do alone. Such AI software can also help banks and financial institutions monitor employees behavior to see if they are in line with regulations.
For instance, AI vendor Digital Reasoning offers Financial Conduct Surveillance. Conduct Surveillance is a natural language processing-based software that analyzes text and voice to determine whether or not a bank’s employees are acting within compliance rules. In another use-case the software might be used to identify which compliance conduct alerts that banks are receiving are relevant and need action.
In a case study, Digital Reasoning claims they helped a bank reduce false positive compliance alerts created by a bank’s existing system. The bank was receiving over 8,000 alerts generated by their existing legacy software tool CA Data Protection, which was leaving the bank’s compliance analysts with little time to thoroughly inspect incidents in more detail.
Digital reasoning claims their software helped read through all the generated alerts and prompted analysts only in cases which were relevant and needed human intervention. The company claims this as a 250% increase in “analytics acuity,” which they do not elaborate on. The company also claims their software helped reduce the time taken in the task and managed to monitor 100% of all the alerts generated daily with a satisfactory level of detail.
Lending
Banks and other financial institutions have used AI in the past for identifying which of their customers might default on credit card payments. Banks collect a lot of information about their customers, including their account and transaction details, their geolocations, their past interactions with the bank, and so on.
AI software could help banks use all of this information and information such as social media data to arrive at an accurate credit rating for each customer. In the past, banks were not able to capitalize on adding customers with little or no credit history to their lending products.
Companies like ZestFinance offers ways for banks to improve their risk-mitigation by allowing for a more complete profile of each customer, indicating the likelihood of default from that customer. AI software could help automate the process and help loan officers assess and monitor far more customer loan applications without increasing the risk associated with adding new customers.
For instance in a case study, ZestFinance claims to have worked alongside Ford Motor Credit Company in a pilot project aimed at applying machine learning to better risk forecasting and monitoring applications in auto financing. The project was also focused on targeting millennial customers and customers with limited credit history information.
The company’s software reportedly uses several streams of data such as news and social media and assign a credit score for each potential customer. ZestFinance claims Ford Credit is planning to invest in machine learning-based credit approval models in the future.
Key Takeaways for Banking Leaders
- Some risk monitoring processes are a natural fit for machine learning. For example, finding outlier patterns among large sets of data (anomaly detection) is one of the strengths of machine learning. Banks may want to consider anomaly detection for their fraud and cybersecurity processes.
- Banks are already investing in automation for risk-related functions. AI could augment the capabilities of fraud investigators and compliance teams to assess a large amount of data at scale.
- In lending, AI systems can help banks make use of vast amounts of customer data to process loan applications faster, assess the creditworthiness of an individual, or help with increasing numbers of customers, keeping risk levels more or less the same.
- Banks might need to build their capabilities in handling data and several of the top 100 banks claim they are engaged in AI projects dealing with the collection an analysis of financial data, such as trading histories or portfolio/company performance figures. This type of AI-enabled risk-mitigation in trading and investing applications are also an area where banks can augment the skills of their employees.
To read more about artificial intelligence we recommend you to read this: How artificial intelligence is fighting financial crime